【#文档大全网# 导语】以下是®文档大全网的小编为您整理的《多层径向基函数网络-4》,欢迎阅读!
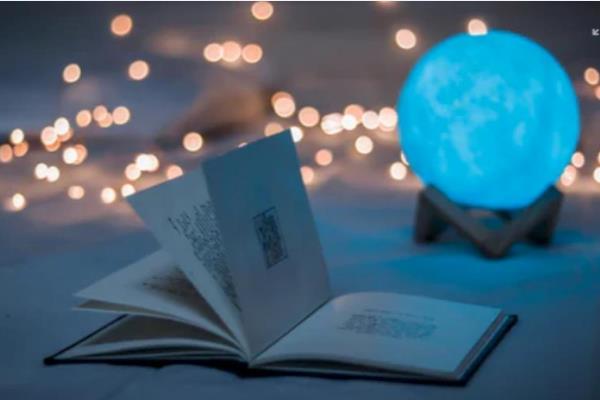
┊ ┊ ┊ ┊ ┊ ┊ ┊ ┊ ┊ ┊ ┊ ┊ ┊ 装 ┊ ┊ ┊ ┊ ┊ 订 ┊ ┊ ┊ ┊ ┊ 线 ┊ ┊ ┊ ┊ ┊ ┊ ┊ ┊ ┊ ┊ ┊ ┊ ┊
安徽工业大学 毕业论文
第一章 引 言
随着中国资本市场的迅速发展和居民收入水平的较大提高,越来越多的人参与到股票市场的投资中,希望能够实现财富的保值和增值。虽然投资股票可能带来高回报,但是同时又蕴含着高风险,其时刻变化不定的价格,让投资者感受到这个市场的复杂性,所以他们迫切需要一种用来解释价格变动原因的理论,最好有一种科学的预测方法来指导投资,从而规避或在锁定风险的同时,获得最大的投资回报。股票价格的预测是一个世界性难题,但这项研究却蕴含了巨大的潜在商业价值,所以吸引着全球许多的学者专家对其进行探索和研究。
在承认股票价格走势可以被预测的基础上,本文阐述了用来预测股票价格走势的各种方法,既包括常规的的基本面分析和技术分析等方法,也包括基于时间序列分析的多层径向函数神经网络这种现代预测方法,当然由于影响股票价格的因素有很多种,一种简单的模型或方法是不能够完全预测其未来的涨跌情况的。本文尝试使用多层径向函数神经网络模型和SAS统计分析软件的强大功能的结合对股价波动趋势进行短期预测,发现在短期预测上有良好的效果,这对提醒投资者注意风险和帮助投资者把握买点和卖点上有较强的现实意义和一定的实用价值。
一、 股票价格预测的背景分析
股票市场与国民经济发展密切相关,是金融市场的重要组成部分,股票作为资本证券化的工具,是虚拟资本中比较普遍的一种,上市公司可以方便地通过股票市场进行融资活动实现套利或者追加项目的投入资金等。从表面上看,股票市场的运行是缺乏秩序的,或者说通常意义下是没有规律性可言的,似乎指数及股价的变动是难以预测的。在资本市场中有许多经济学家用倾其一生的精力,研究出各种数学模型来研究股票市场,试图定量地去把握资本的流动方向及其规律性。
自各种金融市场和股票市场的诞生,人们对以股票价格为代表的各种金融资产价格的分析预测方法的研究就没有停止过,这其中涌现出了各种各样的分析方法体系。虽然这些方法派系众多,比如:基本分析流派、技术分析流派、学术分析流派和行为分析流派等等,但从出发点和分析对象来看,可以分为基本分析(fundamental analysis)和技术分析(technical analysis)两大类。基本分析是依照价格围绕价值波动的原理,通过收集相关的数据和资料,研究影响证券价格的各种基本因素,确定证券的内在价值,找出被市场错误定价的证券,买入并长期持有,直到证券的市场价格回归到它的内在价值为止。技术分析则是根据证券市场信息的历史数据,通过图表、技术指标等探求股票价格变化的内在规律,从而找出最佳的买入点和卖出点。
随着各种数据分析工具开发和数学理论研究的深入,人们用各种不同的方法和工具来分析金融时间序列,做出各种金融时间序列预测的模型,特别是在股票价格的预测模型方面更是数不胜数。时间序列分析方法是统计学研究的一个重要分支,以事物在不同时刻所形成的数据作为研究对象,通过对时间序列数据的特征进行分析和研
共 44 页 第 1 页
┊ ┊ ┊ ┊ ┊ ┊ ┊ ┊ ┊ ┊ ┊ ┊ ┊ 装 ┊ ┊ ┊ ┊ ┊ 订 ┊ ┊ ┊ ┊ ┊ 线 ┊ ┊ ┊ ┊ ┊ ┊ ┊ ┊ ┊ ┊ ┊ ┊ ┊
安徽工业大学 毕业论文
究,探索事物的发展变化规律。经典的时间序列分析方法有图表法、指标法和模型法本文主要取用模型法。模型法是当前对时间序列进行深层次分析和刻画的主要方法,利用事物过去和现在的变化规律数据,构造依时间变化的序列模型,借助外推原则来推测未来是模型法的分析思路。时间序列模型分析方法具有逻辑的美感,模型的形式通常是一个或者一组数学方程。这既具有表达上的简洁性,又可在解释性和可理解性有非常好的效果,为进一步的处理、推导和应用提供方便。
二、传统预测分析方法
传统的预测分析方法主要是指基本分析方法和技术分析方法,这是目前仍然被广大投资者广泛使用的方法。股票的市场价格由它的价值决定,但同时也受到许多因素的影响。其中,供求关系是最直接的因素,其他因素都是通过作用于供求关系而影响股票价格的。
(一) 基本分析方法
1.基本面分析的基本原理
基本面分析一般是指对宏观经济面、公司主营业务所处行业、公司业务同行业竞争水平和公司内部管理水平包括对管理层的考察这诸多方面的分析,数据在这里充当了最大的分析依据,但往往不能以数据来做最终的投资决策,如果数据可以解决问题,那计算机早就代替人脑完成基本面分析,事实上除了数据还要包括许许多多无法以数据来衡量的东西,比如商誉、垄断、管理层人员素质等许多方面难以用数据来表达,这些方面的东西相对而言就成了分析中比较主观的成分。证券分析师根据经济学、金融学、财务管理学及投资学等基本原理,对决定证券价值及价格的基本要素,如宏观经济指标、经济政策走势、行业发展状况、产品市场状况、公司销售和财务状况等进行分析,评估证券的投资价值,判断证券的合理价位,提出相应的投资建议。
基本面分析重点研究股票的内在价值也即是上市公司经营状况的真实反映。这种分析方法认为股票价格在市场上的频繁波动是受多种内外因素影响的,但股票的市场价格总是围绕着内在价值上下波动,市场价格和内在价值之间的差距最终会被市场纠正,即回归价值。因此市场价格低于(或高于)内在价值之时,便是买(卖)机会到来之时。影响股票市场价格波动的因素很多,主要的有三个方面的原因:一是宏观因素为国家经济的繁荣或萧条,二是中观方面的企业所处行业以及相关行业的发展状况,三是微观的角度看是企业自生的经营状况,如经营不当,盈利能力比较弱,则它的股票价值就低,价格就低,反之,则价值就高,价格就贵。 2. 基本分析应考虑主要因素
由于影响股票价格的因素复杂多变,所以股票的市场价格呈现出高低起伏的波动性特征。基本分析法通过对决定股票内在价值和影响股票价格的宏观经济形势、行业状况、公司经营状况等进行分析,评估股票的投资价值和合理价值,与股票市场价进行比较,相应形成买卖的建议。基本分析包括下面三个方面内容:
(1)宏观经济分析。研究经济政策(货币政策、财政政策、税收政策、产业政策等等)、经济指标(国内生产总值、失业率、通胀率、利率、汇率等等)对股票市场
共 44 页 第 2 页
┊ ┊ ┊ ┊ ┊ ┊ ┊ ┊ ┊ ┊ ┊ ┊ ┊ 装 ┊ ┊ ┊ ┊ ┊ 订 ┊ ┊ ┊ ┊ ┊ 线 ┊ ┊ ┊ ┊ ┊ ┊ ┊ ┊ ┊ ┊ ┊ ┊ ┊
安徽工业大学 毕业论文
的影响。
(2)行业分析。分析产业前景、区域经济发展对上市公司的影响 (3)公司分析。具体分析上市公司行业地位、市场前景、财务状况
(二) 技术分析的方法体系
在价、量历史资料基础上进行的数学计算、统计和绘制图表的方法是技术分析方法的主要手段。技术分析的方法繁多,形式多样,本章仅介绍一些比较实用和比较常用的技术分析方法。一般说来,可以将技术分析方法分为如下五类:指标类、K线类、切线类、波浪类、形态类。
1 指标类
指标类要考虑市场行为的各个方面,建立一个数学模型,给出数学上的计算公式,得到一个体现股票市场的某个方面内在实质的数字,这个数字叫指标值。指标值的相互间关系和具体数值,直接反映股票市场所处的状态,为我们的操作行为提供指导方向。指标所反映出来的许多信息是不能从行情报表信息中直接看出来的。 2 K线类
K线类的研究手法是侧重若干天的K线组合情况,推测股票市场多空双方力量的对比,进而判断股票市场多空双方谁占优势,是暂时的,还是决定性的。K线图是进行各种技术分析的最重要的图表。
3 切线类
切线类是按一定方法和原则在由股票价格的数据所绘制的图表中画出一些直线,然后根据这些直线的情况推测股票价格的未来趋势,这些直线就叫切线。切线的作用主要是起支撑和压力的作用。支撑线和压力线的往后延伸位置对价格趋势起一定的制约作用。 4 波浪类
波浪理论把股价的上下变动和不同时期的持续上涨、下跌看成是波浪的上下起伏。波浪的起伏遵循自然界的规律,股票的价格运动也就遵循波浪起伏的规律。波浪理论较之于别的技术分析流派,最大的区别就是能提前很长的时间预计到行情的底和顶,而别的流派往往要等到新的趋势已经确立之后才能看到。但是,波浪理论又是公认的较难掌握的技术分析方法。 5 形态类
形态类是根据价格图表中过去一段时间走过的轨迹形态来预测股票价格未来趋势的方法。根据技术分析的假设,市场行为包括了一切信息。价格走出的形态是市场分析的重要部分,是股票市场对各种信息进行消化后的具体表现。从而用价格的形态来推测股票价格的将来就十分有意义。
三、 技术分析的发展趋势
在20世纪70年代曾有人提出了著名的有效市场假设,认为股票价格的波动是随机游走过程,未来的价格是不可能被预测的,人们不可能依靠分析公开信息和历史价格信息来获得超额收益。然而,近年来越来越多的实践和研究表明,这种理论与事实
共 44 页 第 3 页
┊ ┊ ┊ ┊ ┊ ┊ ┊ ┊ ┊ ┊ ┊ ┊ ┊ 装 ┊ ┊ ┊ ┊ ┊ 订 ┊ ┊ ┊ ┊ ┊ 线 ┊ ┊ ┊ ┊ ┊ ┊ ┊ ┊ ┊ ┊ ┊ ┊ ┊
安徽工业大学 毕业论文
是不符合的。为了更充分地解释证券市场的运动规律,在20世纪90年代初又有学者提出了分形市场假说,强调证券市场投资时间尺度和信息接受程度对投资者行为的影响,认为所有稳定的市场都存在着分形结构,证券价格走势在一定程度上是可以被预测的。
近十几年来,证券分析技术出现了一些新的发展趋势。计算机技术的进步使各种分析、预测理论得以和信息技术、统计学、优化算法紧密结合,使证券分析向数量化、技术化、复杂化方向发展。将随机过程,混沌理论、模糊数学等数学理论和神经网络、人工智能等工程学手段应用于股票价格预测逐渐成为了现在股票价格预测分析的热门领域。西方金融理论界对技术分析的研究主要集中于两个方面:寻求价格运动规律存在的统计支持:研究基于技术分析的交易规则,获得更直接的实证支持。比如在统计上对证券收益中厚尾现象(fat tail)的发现,使得一些研究者采用分形和混沌理论来解释证券的价格行为,并得到很好的效果。还有贝叶斯统计、神经元网络、人工智能等方法,这些工程学的手段也逐步使用在各种投资软件和自动交易系统中。与简单的移动平均等方法相比,现代技术分析在预测技术方面达到了更高的境界。
第二章 文献综述
预测是根据过去和现在估计未来。实际资料是预测的依据,科学理论是预测的基础,数学模型是预测的手段。神经网络用于股价预测,与传统方法相比具有很大的优越性。它不需要事先知道被控对象的模型,就可以实现任意的函数关系、学习能力以及通过学习掌握数据之间的依存关系。并且它只需要通过对历史数据的学习,就能够从纷繁复杂的数据中自主寻找出参数之间的规律和特点,并且刻画这些规律和特点。目前,根据所使用的神经网络结构类型的不同,大致可以分为六类:
一、误差反传神经网络(Back-propagation Neural Network )
误差反传神经网络也称为多层前向网络,简称BP 神经网络,是目前在股票预测中应用最广泛的一种神经网络。文[1]利用BP 神经网络和公司在各个层面的财务指标来预测上市公司的股价;文[2]采用改进BP 算法的误差反传神经网络对综合指数的收盘价及个股的收盘价、股票价格变化趋势进行了预测,并与ARIMA 预测模型的结果进行了比较,结果表明神经网络在股价变化趋势方面优于ARIMA 预测模型;文[3-5]提出BP 神经网络与遗传算法结合的股价预测模型,对部分大股和个股的收盘价进行预测,验证了该预测模型的有效性。
二、模糊神经网络(Fuzzy Neural Network)
由于模糊神经网络(简称FNN)处理非线性、模糊性等问题上的优越性,以及它在智能信息处理方面拥有的巨大潜力,使得越来越多的专家学者投入到这个领域中来,并做出了卓有成效的研究成果。文[6]提出了一个面向股市预测的模糊神经网络系统,对某上市股票进行了短期预测,结果表明该方法对股票市场的短期预测是可行的和有
共 44 页 第 4 页
┊ ┊ ┊ ┊ ┊ ┊ ┊ ┊ ┊ ┊ ┊ ┊ ┊ 装 ┊ ┊ ┊ ┊ ┊ 订 ┊ ┊ ┊ ┊ ┊ 线 ┊ ┊ ┊ ┊ ┊ ┊ ┊ ┊ ┊ ┊ ┊ ┊ ┊
安徽工业大学 毕业论文
效的;文[7]利用股票市场的理论和模糊神经网络进行预测股票价格;文[8]利用模糊集理论和模糊概率的方法来模拟股价分析过程和研究投资策略,由此获得了一些有关投资决策的关键技术指标和控制风险的策略。
三、 小波神经网络(Wavelet Network Network)
小波分析理论与神经网络结合形成小波神经网络(简称WNN)。它将小波分析良好的时频局部性质和神经网络自学习功能及良好的容错能力有效的结合起来[9]。文[10,11]以小波神经网络建立预测模型对个股进行预测,取得了较为满意的结果;在文献[12]中,利用遗传算法对小波包进行最优小波包分解的选择,并训练神经网络,对股指的走势进行预测,取得了比较满意的预测效果。
四、 径向基函数神经网络(Radial-Basis Function Neural Network)
径向基函数神经网络简称RBFNN,由于这种网络类型不存在局部最优的问题,使得其在非线性时间序列预测中得到广泛的应用。文[13]以开盘价、收盘价、最高价、最低价作为神经网络的输入变量,利用RBFNN自适应算法建立起神经网络模型,以当天的股票平均价格作为预测对象;文献[14]把RBFNN与混沌理论结合,以股票的实际收盘价作为预测对象,建立了基于RBFNN的股市混沌预测模型,结果表明用RBFNN预测股价是可行的的和有效的;文[15]借助RBFNN对非线性函数的逼近能力,对深能源A股价作了连续若干天的一步预测,也收到了比较好的预测结果。
五、 回归神经网络(Recurrent Neural Network)
回归神经网络也称为递归神经网络,简称RNN,其网络结构与其它神经网络结构的不同之处在于它多了一个反馈层,这使得它具有动态记忆的特点。文献[16]使用动态递归神经网络—标准Elman 模型的一种变形模型(输入层与隐层均加入反馈层)建立预测模型,以五个不同股票市场(加拿大、香港、英国、美国、日本)的历史交易数据作为样本,预测下一年的股票回报,为个人投资决策提供参考,做出了成功的探索;文[17]采用快速BP 算法的递归神经网络——标准Elman 模型对股价做出预测,结果表明对于比较稳定的股票数据,采用快速BP 算法的标准Elman 模型的预测结果比多层前馈网络、径向基函数网络的预测结果要好;文献[18]采用BP 算法的标准
六、 概率神经网络
概率神经网络是在RBFNN 和窗函数密度估计方法的基础上提出的一种新的人工神经网络。它利用了样本的先验概率—Bayes 定律和最优判定原则对新的样本进行分类, 其不受多元正态分布等条件的限制,并且在运算过程中可算出被分类的新输入样本的后验概率,从而提供对计算结果的解释。目前,它在股票预测方面的应用不多。文献[19]分别运用五个模型:概率神经网络、径向基函数神经网络、径向基函数概率混合神经网络、EM 算法优化的径向基函数概率混合神经网络、遗传算法优化的径向基函数概率混合神经网络,建立对股票波动率的预测模型,得到了令人满意的实验结果。用神经网络对股市预测,预测对象也多种多样。目前主要有以下五种预测对象:当日股指预测、股价趋势预测、股票投资策略、股票系统风险预测和股票收益预测,还有对股票内在价值的预测[20]等等。本文以股票未来两天内的最高价作为预测对
共 44 页 第 5 页
┊ ┊ ┊ ┊ ┊ ┊ ┊ ┊ ┊ ┊ ┊ ┊ ┊ 装 ┊ ┊ ┊ ┊ ┊ 订 ┊ ┊ ┊ ┊ ┊ 线 ┊ ┊ ┊ ┊ ┊ ┊ ┊ ┊ ┊ ┊ ┊ ┊ ┊
安徽工业大学 毕业论文
象。
近年来,径向基网络(RBF)仍是人工神经网络研究的热点之一,每年都有大量的成果出现,包括理论研究,算法设计,以及大量的各个学科领域的应用,包括模式识别和图象处理、自动控制、函数逼近、时间序列、数据挖掘,通信等领域。因此RBF 网络的研究具有巨大的理论价值和实用价值。RBF 网络的最大优点是其具有很强的函数逼近能力。理论证明只要RBF 网络的隐节点足够多,则它可以以任意精度逼近紧集上的连续函数。但是如何提高和改进RBF 网络的性能仍需要深入研究。现有的RBF 网络的学习策略主要集中在RBF 网络中心的确认,即在径向基函数数目预先确定的条件下,RBF 的中心从训练集中随机的选取或由训练集以某种无监督方式或有监督方式确定。这就产生了像多层前馈神经网络一样如何确定隐层节点个数的问题,它常常导致网络具有较差的性能和较大的不必要的体积,并且增加网络隐层节点个数,不能较好地改善网络的性能,从而造成网络资源的浪费,而RBF 函数中心的选择显然也是不能令人满意的。
第三章 多层径向函数网络
一、单层径向函数网络算法综述
单层径向神经网络采用高斯函数等径向函数作为神经元传递函数,用于非线性函数的逼近。从网络结构上看 , 它是由第一层的输入层,第二层的隐层和第三层的输出层组成。输入层是由输入样本节点组成;隐层为径向基层,作用函数是径向函数,当输入样本落到输入空间某一小范围里时,隐层才会发挥他的作用,隐层的输出结果是各隐层的加权和,从而完成从输入空间到隐含层空间的非线性变换;输出层为线性层,提供从隐层空间到输出层空间的线性变换。 图1说明了径向神经网络的整个输入输出过程。
图1 径向函数输入输出过程
径向基函数网络的输入输出关系可以表示成如下形式
f(x)wiixci,i
i1m
共 44 页 第 6 页
┊ ┊ ┊ ┊ ┊ ┊ ┊ ┊ ┊ ┊ ┊ ┊ ┊ 装 ┊ ┊ ┊ ┊ ┊ 订 ┊ ┊ ┊ ┊ ┊ 线 ┊ ┊ ┊ ┊ ┊ ┊ ┊ ┊ ┊ ┊ ┊ ┊ ┊
安徽工业大学 毕业论文
这里ck,k是径向函数参数,其中ck是径向函数的中心,k为径向函数的宽度参数。径向函数网络的学习有以下几种典型方法
(a) Mood和Darken的算法[21]
这个算法先对样本的输入部分做聚类分析,通常是k-mean法,将样本的输入部分分成若干类,算出每类的重心作为径向函数的中心,再指定径向函数的宽度参数,然后用最小二乘法确定各径向函数的组合系数。
(b) Poggio算法[22]
和Mood和Darken的算法不同,这个算法将每个样本的输入部分作为一个径向函 数的中心,这样径向函数的个数就是样本的个数。然后指定径向函数的宽度参数,再用最小二乘法确定各径向函数的组合系数。
(c) 正则正交最小二乘法[23]
N
设样本为yk,xkk1。每个样本输入都是径向函数基的中心的候选。记
cixi,
1iN
ikixkci,i
当所有样本输入都被选为基函数的中心是,有
y(k)iikek,1kN
i1N
记
T
yy1,y2,,yN
T
1,2,,N T
ee1,e2,,eN
1,2,,N i11,22,,NN
则得矩阵表现形式如下
ye
将矩阵分解为正交回归矩阵形式WA,则有
yWge
这里g满足gA。当xi被选为径向函数基的中心时,模型的逼近误差下降率为
rerriwiTwigi2yTy
可以选对下降率贡献大的输入样本,直到
共 44 页 第 7 页
┊ ┊ ┊ ┊ ┊ ┊ ┊ ┊ ┊ ┊ ┊ ┊ ┊ 装 ┊ ┊ ┊ ┊ ┊ 订 ┊ ┊ ┊ ┊ ┊ 线 ┊ ┊ ┊ ┊ ┊ ┊ ┊ ┊ ┊ ┊ ┊ ┊ ┊
安徽工业大学 毕业论文
1rerri
i1
Ns
被满足是停止。这里为选定的容许误差。这时网络的径向函数的个数即为Ns。 (d) 局部学习法[24]
局部学习法的径向函数都选用这样的函数,它们在各自的一个局部空间上不为0,而其余空间上都为0. 这样,这些径向函数都是接近正交的。于是我们可以一个一个地把径向函数加到网络上去,直到拟合误差小于预先确定的值为止。
在以上的各方法中,Mood和Darken的算法是最基本的,也是最简单的。其它的方法旨在解决径向函数的个数确定问题。近年来发展的方法也是以怎样快速地寻找径向函数的中心,确定径向函数的个数为宗旨而提出来的[25,26,27,28]。这里我们从另一个角度出发,改善Mood和Darken的算法的精度问题,同时间接地解决基函数的个数问题。为此,我们提出了多层径向函数网络。一层径向函数的逼近能力是有限的。在一层网络的基础上用第二层网络去拟合第一层网络的残差函数,这样拟合精度就提高了。再在两层网络的基础上,用第三层网络去拟合两层网络的拟合残差函数,得到了三层网络,它的拟合精度又提高了。如此进行下去,就得到一个高精度的多层径向函数网络。
单层径向函数网络的逼近精度较低,限制了它的应用效果。这里我们提出多层径向函数网络,以克服这个缺点。高精度的函数逼近网络在非线性建模,特别是非线性时间序列和混沌时间序列的建模和预测上有着重要的意义。
二、多层径向基函数网络(Multi-layer Radial Basis Function Network)
(一)径向基函数网络的基本概念
(1)径向基函数(Radial Basis Function) 径向函数指的是峰状型的函数,如高斯函数
xx0
xexp
22这里x,x0为n维的向量,xx0
2
x
1n
xnx01x0
2
2
。
(2) 径向基函数网络(Radial Basis Function Network)
径向函数网络是一组径向函数的线性组合,即
f(x)wkkx
k1m
这里
xx2
k
kxexp 2dk
构造这个网络的目的是用它去逼近一个真实的函数gx。通常gx是不知道的。我们知道的只是有限个样本
共 44 页 第 8 页
┊ ┊ ┊ ┊ ┊ ┊ ┊ ┊ ┊ ┊ ┊ ┊ ┊ 装 ┊ ┊ ┊ ┊ ┊ 订 ┊ ┊ ┊ ┊ ┊ 线 ┊ ┊ ┊ ┊ ┊ ┊ ┊ ┊ ┊ ┊ ┊ ┊ ┊
安徽工业大学 毕业论文
x1,x2,,xN
和它们所对应的函数值
y1,y2,,yN
这里如果
y1,y2,,yN
是确定性的,即
yigxi,i1,2,,N
那么这就是一个函数逼近的问题。如果是不确定性的,即
yigxii,i1,2,,N
这里的i为随机噪声,那么这就是一个非参数回归的问题。
按Mood和Darken的算法这个网络可以按如下步骤构造的。 设数据样本为
x1,x2,,xN
它们所对应的函数值为
y1,y2,,yN
将x1,x2,,xN分成m个聚类(通常用K-平均法)
xk1,xk2,,xkn1ink1km
k
它们的中心被定义为
1xk
nk
令
N
x
i1
nk
ki
1km
RSS(yif(xi))2min
k1
用最小二乘法决定加权系数
w1,w2,,wm
这样得到的就是普通的径向函数网络。 (二) 多层径向基函数网络
假设我们已经得到了单层镜像函数网络
1
f1(x)w1kkx
k1m
这里
xx1
k
1xexpkd112k
2
令
ei1yif1(xi)1iN
共 44 页 第 9 页
┊ ┊ ┊ ┊ ┊ ┊ ┊ ┊ ┊ ┊ ┊ ┊ ┊ 装 ┊ ┊ ┊ ┊ ┊ 订 ┊ ┊ ┊ ┊ ┊ 线 ┊ ┊ ┊ ┊ ┊ ┊ ┊ ┊ ┊ ┊ ┊ ┊ ┊
安徽工业大学 毕业论文
这就是单层镜像函数网络所实现的函数在xi处的值与实际值yi的误差。
做增广样本
1
xi(xi,ei)1iN
这里1。再将
,xx12,,xN
分成m个聚类
1
x(x,e1km kikii)1ink
这样我们就得到了m个新的聚类
xk1,xk2,,xknk
它们的中心是
1km
1
x
nk
2k
x
i1
nk
ki
1km
令
RSS(ei1f2(xi))2min
i1N
用最小二乘法决定加权系数
22
w12,w2,,wm
使
22
f2(x)wkkx
k1m
这里
xx22
k
2xexp k2d22k
也就是说,我们用一个新的径向函数网络去拟合前一层网络的拟合残差
ei1yif1(xi)1iN
于是我们得到了一个更精确的模型
F2(x)f1(x)f2(x)
这就是一个2层镜像函数网络。
重复这一步骤,我们得到一个多层镜像函数网络
共 44 页 第 10 页
┊ ┊ ┊ ┊ ┊ ┊ ┊ ┊ ┊ ┊ ┊ ┊ ┊ 装 ┊ ┊ ┊ ┊ ┊ 订 ┊ ┊ ┊ ┊ ┊ 线 ┊ ┊ ┊ ┊ ┊ ┊ ┊ ┊ ┊ ┊ ┊ ┊ ┊
安徽工业大学 毕业论文
Fk(x)f1(x)f2(x)f3(x)fk(x)
根据适当的判别准则,已决定增加几层最为适合。
需要说明的是,在增广样本
1
xi(xi,ei)1iN
中,我们用ei1代替ei1是因为ei1的绝对值往往远小于xi的各分量的绝对值,这样新的聚类
xk1,xk2,,xknk1ink
受拟合残差
1km
ei1yif1(xi)1iN
的影响就小,所得到的函数f2(x)对残差的抵消作用就小。
三、用多层径向基函数网络做非参数回归
用多层径向基函数网络做非参数回归分析时,本文采用如下方法确定网络的结构
和参数。
1. 网络层数的确定
确定网络层数最简单的方法是将样本分为两组,一组用于学习,另一组用于检验。为简单起见,记用于学习的样本为
x1,x2,,xN
它们所对应的函数值为
y1,y2,,yN
用于检验的样本为
xN1,xN2,,xM
它们所对应的函数值为
yN1,yN2,,yM 对于学习样本,第1层网络的残差为
ei1yif1(xi)1iN
残差平方和RSS1为
RSS1(yif1(xi))2
i1N
对于检验样本,第1层网络的拟合残差为
ei1yif1(xi)N1iM
残差平方和RSS1为
RSS1
iN1
(y
M
i
f1(xi))2
对第2层网络,学习样本和检验样本的拟合残差分别为
共 44 页 第 11 页
┊ ┊ ┊ ┊ ┊ ┊ ┊ ┊ ┊ ┊ ┊ ┊ ┊ 装 ┊ ┊ ┊ ┊ ┊ 订 ┊ ┊ ┊ ┊ ┊ 线 ┊ ┊ ┊ ┊ ┊ ┊ ┊ ┊ ┊ ┊ ┊ ┊ ┊
安徽工业大学 毕业论文
ei2ei1f2(xi)1iN ei2ei1f2(xi)
残差平方和分别为
RSS2(ei1f2(xi))2 RSS2
Ni1
M
N1iM
iN1
(e
1if2(xi))2
如此计算下去,记第k层径向函数网络对学习样本和检验样本的拟合残差分别为
eikeik1fk(xi)1iN
eikeik1fk(xi)N1iM
残差平方和分别为
RSSk(eik1fk(xi))2 RSSk
Ni1
M
iN1
(e
k1ifk(xi))2
那么如果
RSS2RSSRSS1p1RSSpRSSp1
就取p层网络为我们所需的模型。
需要说明的是,在非线性回归和非线性随机时间序列分析中,有时不宜设置检验样本集。我们必须采用信息量准则来确定哪层最佳。但多层径向基函数网络的信息量的计算尚处于研究阶段,所以我们只能用训练集的残差平方和来衡量模型的优劣。虽然这样确定的模型有过拟合的可能,但在许多实际数据的分析中仍得到了高精度的预测。 2.ik的确定
对第i层网络中的第k个聚类,ik可取为该聚类中样本的样本均方,即
2
2
1
nk
2ik
j1
nk
xnjx
ik
2
如果聚类中只有一个样本xp,则取这个聚类的样本方差为该层各聚类样本方差的平均值。
3.确定和d
由试探法决定。一般可取1到10之间。对前几层,因为误差较大,可取得小一些,后几层,因为误差较小,可取得大一些。一般说来取得大,在增广样本的聚类分析中,误差项对聚类的产生的影响也大,拟合精度提高。但是过大,拟合误差不随网络层数的增加而减小甚至增大,所以确定一个合理的值是很有必要的。我们可以用一维搜索来确定的值。即先确定的取值区间,然后搜索,使检验样本的
达到最小。 残差平方和RSSk
d越大,径向基函数越平坦,不会出现过拟合现象,但太平坦了会降低拟合精度。反之,d越小,径向基函数越尖,拟合精度提高,但太尖了,会出现过拟合现象。因
共 44 页 第 12 页
┊ ┊ ┊ ┊ ┊ ┊ ┊ ┊ ┊ ┊ ┊ ┊ ┊ 装 ┊ ┊ ┊ ┊ ┊ 订 ┊ ┊ ┊ ┊ ┊ 线 ┊ ┊ ┊ ┊ ┊ ┊ ┊ ┊ ┊ ┊ ┊ ┊ ┊
安徽工业大学 毕业论文
此确定一个恰当的值是很有重要的。做法之一就是确定一个d的取值区间,对d的一
d,用一维搜索的方法求得使检验样本个取值,计算对检验样本的残差平方和RSSk
d达到最小的最佳值。 的残差平方和RSSk
同时依赖于和d。我们可以按如下的方法然而,检验样本的残差平方和RSSk
最小。探索和d的最佳值。先固定的值,即令1,搜索d。设当dd1时RSSk
最小。在固定2,搜索d。当dd2再固定dd1,搜索。设当2是RSSk
最小。再固定dd2,搜索。如此进行下去,直到RSSk收敛或,d收敛。时RSSk 4.径向基函数个数的确定
每层径向基函数的个数也就是聚类个数。先确定第一层的聚类个数n1。可以通过根据样本个数决定,如取样本数的1/30到1/10,也就是平均每个聚类分配10至30个样本。因为拟合误差函数一般比原函数形状复杂,所以第二层以后的聚类个数可取得大一些,一般nk取n1的1到2倍,但必须保证每个聚类的平均样本个数为5,即nk不超过样本数的1/5。
第四章 时间序列的建模与预测
一、基本原理
用多层径向基函数网络对时间序列进行建模是使用这种网络作非线性回归模 型的特例。时间序列的一般模型为
xn1gxn,xn1,,xnkn1
这里n1为白噪声。令
x1,x1,x2,,xk
为初始条件,对应的输出y的值为
yk1xk,yk2xk1,,yN1xN
于是有
xk1gxk,xk1,,x1k1 xk2gxk1,xk,,x2k2
xn1gxn,xn1,,xnkn1
xNgxN1,xN2,,xNk1N
这样就可以用第三章介绍的方法建模。确定k的过程如下:
共 44 页 第 13 页
┊ ┊ ┊ ┊ ┊ ┊ ┊ ┊ ┊ ┊ ┊ ┊ ┊ 装 ┊ ┊ ┊ ┊ ┊ 订 ┊ ┊ ┊ ┊ ┊ 线 ┊ ┊ ┊ ┊ ┊ ┊ ┊ ┊ ┊ ┊ ┊ ┊ ┊
安徽工业大学 毕业论文
令k1,记模型信息量,如AIC的值为AIC1。再令k2,记模型的记模型信息量,如AIC的值为AIC2。再令k3,记模型信息量,如AIC的值为AIC3。假设
AICm1AICmAICm1
则取km
在完成建模后,就可以对时间序列进行预测。我们从已知的
ˆN1 xNk,xNk1,xNk2,,xN出发,得到xN1的估计值x
ˆN1gxN,xN1,,xNk1 x
再令
ˆN2gxˆN1,xN,,xNk x
ˆN3gxˆN2,xˆN1,,xNk x
ˆNk1gxˆNk,xˆNk1,,xˆN1 x
ˆN11gxˆN1,xˆN11,,xˆN1k1 x
由此,我们得到了一组时间序列的预测值
ˆN1,xˆN2,,xˆN11。 x
二、在股票预测中的应用
本文选用了中视传媒(600088)自2009年4月20日到2010年6月10日的股票
收盘价格数据,共280个数据。在试探过程中设置程序的最大层数为20。样本个数n=280,将数据分为两组,一组用于训练学习,另一组作为预测,其中275个数据用作学习,5个数据作为预测,通过我们试探,增广数据系数取5比较适宜。本文采取将延迟步数定为从1到5,在每一类延迟步数中通过试探,选出最佳的宽度系数d值,再对这5种类型的拟合精确度进行比较,最终得到最佳模型。需要说明的是,多层径向基函数网络的信息量的计算公式还在研究阶段,我们暂时用残差平方和RSS代替信息量。这样做可能会使模型产生过拟合现象,使预测精度降低,但计算机实验结果表明模型仍有很好的预测精度。
(一)延迟步数为1的d值确定过程
我们取用于观测样本个数为n275,预测个数为5个,增广数据系数5,第一层聚类数为9,第二次以后的聚类个数为15。宽度系数d通过试探确定。试探结果如下表所示:
共 44 页 第 14 页
┊ ┊ ┊ ┊ ┊ ┊ ┊ ┊ ┊ ┊ ┊ ┊ ┊ 装 ┊ ┊ ┊ ┊ ┊ 订 ┊ ┊ ┊ ┊ ┊ 线 ┊ ┊ ┊ ┊ ┊ ┊ ┊ ┊ ┊ ┊ ┊ ┊ ┊
安徽工业大学 毕业论文
表1 延迟步数为1时d值探求过程(1)
真实值 d=10 d=15 d=20 d=25 d=30
20100604 24.8 24.6914 24.7017 24.7863 24.7696 24.6968 0.44% 0.40%
相对误差
0.06% 0.12% 0.42%
20100607 24.18 24.729 24.7406 24.8448 24.8166 24.7429 2.27% 2.32% 2.75% 2.63% 2.33%
20100608 24.68 24.3324 24.3241 24.2762 24.3118 24.306 1.41% 1.44% 1.64% 1.49% 1.52%
20100609 24.89 24.6827 24.6925 24.7724 24.7582 24.6864 0.83% 0.79% 0.47% 0.53% 0.82%
20100610 25.28 24.7547 24.7665 24.8803 24.8435 24.775 2.08% 2.03% 1.58% 1.73% 2.00%
平均值 24.766 24.63804 24.64508 24.712 24.69994 24.64142 1.406% 1.396% 1.300% 1.300% 1.418%
通过对上表的观察,我们可以发现随着d从10到30的变化,相对误差的平均值呈先减小后增大的变化,其中当d20和d25时取得最小的平均相对误差,这样,我们再通过观测d20至25之间的相对误差的平均值的变化从其中挑选出最佳的d值,试探过程如表2所示:
表2 延迟步数为1时d值探求过程(2)
真实值 d=20 d=21 d=22 d=23 d=24 d=25
20100604 24.8 24.7863 24.797 24.7309 24.7942 24.7918 24.7696 0.0552% 0.0121% 0.2786% 0.0234% 0.0331% 0.1226%
20100607 24.18 24.8448 24.8755 24.775 24.8506 24.8442 24.8166 2.7494% 2.8763% 2.4607% 2.7734% 2.7469% 2.6328%
20100608 24.68 24.2762 24.2397 24.3154 24.2846 24.2938 24.3118 1.6361% 1.7840% 1.4773% 1.6021% 1.5648% 1.4919%
20100609 24.89 24.7724 24.7792 24.7205 24.7807 24.7791 24.7582 0.4725% 0.4452% 0.6810% 0.4391% 0.4456% 0.5295%
20100610 25.28 24.8803 24.9273 24.8025 24.8836 24.874 24.8435 1.5811% 1.3952% 1.8888% 1.5680% 1.6060% 1.7267%
平均值 24.766 24.712 24.72374 24.66886 24.71874 24.71658 24.69994 1.2989% 1.3026% 1.3573% 1.2812% 1.2793% 1.3007%
相对误差m
从图中不难看出,当d24时,相对误差m1.2793%为最小。由此我们可以确定,当5,d24时函数模型的预测精确度最高。这时最佳层数为3。
共 44 页 第 15 页
┊ ┊ ┊ ┊ ┊ ┊ ┊ ┊ ┊ ┊ ┊ ┊ ┊ 装 ┊ ┊ ┊ ┊ ┊ 订 ┊ ┊ ┊ ┊ ┊ 线 ┊ ┊ ┊ ┊ ┊ ┊ ┊ ┊ ┊ ┊ ┊ ┊ ┊
安徽工业大学 毕业论文
表3 延迟步数为1,5 d24时的拟合误差指标
层数 最小误差值 最大误差值 均值误差 残差平方和 ----------------------------------------------------------------------- 1 0.0015654 2.1390533 0.4942203 112.2804893 2 0.000366952 1.9702120 0.4150932 90.5169204 3 0.000228606 1.9704613 0.4151024 90.5169031 4 0.000228606 1.9704613 0.4151024 90.5169031 5 0.000228606 1.9704613 0.4151024 90.5169031 6 0.000228606 1.9704613 0.4151024 90.5169031 7 0.000228606 1.9704613 0.4151024 90.5169031 8 0.000228606 1.9704613 0.4151024 90.5169031 9 0.000228606 1.9704613 0.4151024 90.5169031 10 0.000228606 1.9704613 0.4151024 90.5169031 11 0.000228606 1.9704613 0.4151024 90.5169031 12 0.000228606 1.9704613 0.4151024 90.5169031 13 0.000228606 1.9704613 0.4151024 90.5169031 14 0.000228606 1.9704613 0.4151024 90.5169031 15 0.000228606 1.9704613 0.4151024 90.5169031 16 0.000228606 1.9704613 0.4151024 90.5169031 17 0.000228606 1.9704613 0.4151024 90.5169031 18 0.000228606 1.9704613 0.4151024 90.5169031 19 0.000228606 1.9704613 0.4151024 90.5169031 20 0.000228606 1.9704613 0.4151024 90.5169031
表4 延迟步数为1,5 d24时的预测误差指标 层数 最小误差值 最大误差值 均值误差 残差平方和 ----------------------------------------------------------------------- 1 0.0211526 0.8210685 0.3130287 0.9146263 2 0.0079583 0.6645090 0.3150105 0.7676239 3 0.0081913 0.6642220 0.3151052 0.7675445 4 0.0081913 0.6642220 0.3151052 0.7675445 5 0.0081913 0.6642220 0.3151052 0.7675445 6 0.0081913 0.6642220 0.3151052 0.7675445 7 0.0081913 0.6642220 0.3151052 0.7675445 8 0.0081913 0.6642220 0.3151052 0.7675445 9 0.0081913 0.6642220 0.3151052 0.7675445 10 0.0081913 0.6642220 0.3151052 0.7675445 11 0.0081913 0.6642220 0.3151052 0.7675445 12 0.0081913 0.6642220 0.3151052 0.7675445 13 0.0081913 0.6642220 0.3151052 0.7675445 14 0.0081913 0.6642220 0.3151052 0.7675445 15 0.0081913 0.6642220 0.3151052 0.7675445 16 0.0081913 0.6642220 0.3151052 0.7675445 17 0.0081913 0.6642220 0.3151052 0.7675445 18 0.0081913 0.6642220 0.3151052 0.7675445 19 0.0081913 0.6642220 0.3151052 0.7675445 20 0.0081913 0.6642220 0.3151052 0.7675445 -----------------------------------------------------------------------
通过观察,不难发现当层数从1-20层的变化情况是:随着层数的不断递增,平
RSS由0.9146263逐渐减少至0.7675445,均误差m由0.3130287变大至0.3151052,
共 44 页 第 16 页
┊ ┊ ┊ ┊ ┊ ┊ ┊ ┊ ┊ ┊ ┊ ┊ ┊ 装 ┊ ┊ ┊ ┊ ┊ 订 ┊ ┊ ┊ ┊ ┊ 线 ┊ ┊ ┊ ┊ ┊ ┊ ┊ ┊ ┊ ┊ ┊ ┊ ┊
安徽工业大学 毕业论文
以上两个误差的值到了第三层次之后就不再改变,因此,我们可以认为该网络到此收敛。
延迟步数为1时拟合曲线和预测曲线由图2和图3给出。
图2 延迟步数为1,5,d24时第3层的拟合结果
(星为实际值,点为拟合值)
图3 延迟步数为1,5,d24时第3层的预测结果
(星为实际值,点为拟合值)
共 44 页 第 17 页
┊ ┊ ┊ ┊ ┊ ┊ ┊ ┊ ┊ ┊ ┊ ┊ ┊ 装 ┊ ┊ ┊ ┊ ┊ 订 ┊ ┊ ┊ ┊ ┊ 线 ┊ ┊ ┊ ┊ ┊ ┊ ┊ ┊ ┊ ┊ ┊ ┊ ┊
安徽工业大学 毕业论文
从图中可以很直观的发现,训练集和预测集的拟合程度都非常的高。预测值,误差及相对误差列于表5。由表可以看出相对误差是较小的。
表5 延迟步数为1的预测误差指标
最优参数
时间 20100604
a=5 d=24 层数=3
20100607 20100608 20100609 20100610
收盘价 24.8 24.18 24.68 24.89 25.28
预测收盘价 24.7918 24.8442 24.2938 24.7791 24.874
误差 0.0082 -0.6642 0.3862 0.1109 0.406
相对误差 0.033% 2.747% 1.565% 0.446% 1.606%
(二) 延迟步数为2至5时宽度系数d值的确定
延迟步数为2-5时确定宽度系数d值的过程与延迟步数为1时是一样的,均采用试探的方法,从大范围搜索到小范围搜索。通过这样的试验,可以确定在参数5,延迟步数为2至5的条件下d的最优值。以下列出实验结果
1.当延迟步数为2时的实验结果: 表6给出延迟步数为2时的预测误差指标
表6 延迟步数为2的预测误差指标
最优参数
时间 20100604 20100607
a=5
d=80 20100608 层数=5
20100609
20100610
收盘价 24.8 24.18 24.68 24.89 25.28
预测收盘价 24.065 24.5638 24.6091 24.5093 24.5582
误差 0.735 -0.3838 0.0709 0.3807 0.7218
相对误差 2.964% 1.587% 0.287% 1.530% 2.855%
共 44 页 第 18 页
┊ ┊ ┊ ┊ ┊ ┊ ┊ ┊ ┊ ┊ ┊ ┊ ┊ 装 ┊ ┊ ┊ ┊ ┊ 订 ┊ ┊ ┊ ┊ ┊ 线 ┊ ┊ ┊ ┊ ┊ ┊ ┊ ┊ ┊ ┊ ┊ ┊ ┊
安徽工业大学 毕业论文
图4和图5分别为延迟步数为2时的拟合曲线图和预测曲线图。
图4 延迟步数为2,5,d80时第3层的拟合结果
(星为实际值,点为拟合值)
共 44 页 第 19 页
┊ ┊ ┊ ┊ ┊ ┊ ┊ ┊ ┊ ┊ ┊ ┊ ┊ 装 ┊ ┊ ┊ ┊ ┊ 订 ┊ ┊ ┊ ┊ ┊ 线 ┊ ┊ ┊ ┊ ┊ ┊ ┊ ┊ ┊ ┊ ┊ ┊ ┊
安徽工业大学 毕业论文
图5 延迟步数为2,5,d80时第3层的预测结果
(星为实际值,点为拟合值)
2.当延迟步数为3时的实验结果: 表7给出延迟步数为3时的预测误差指标
表7 延迟步数为3的预测误差指标
最优参数
时间 20100604
a=5 d=50 层数=1
20100607 20100608 20100609 20100610
收盘价 24.8 24.18 24.68 24.89 25.28
预测收盘价 24.405 24.8998 24.7393 25.0123 24.8681
误差 0.395 -0.7198 -0.0593 -0.1223 0.4119
相对误差 1.593% 2.977% 0.240% 0.491% 1.629%
延迟步数为3的拟合曲线和预测曲线由图6和图7给出。
共 44 页 第 20 页
┊ ┊ ┊ ┊ ┊ ┊ ┊ ┊ ┊ ┊ ┊ ┊ ┊ 装 ┊ ┊ ┊ ┊ ┊ 订 ┊ ┊ ┊ ┊ ┊ 线 ┊ ┊ ┊ ┊ ┊ ┊ ┊ ┊ ┊ ┊ ┊ ┊ ┊
安徽工业大学 毕业论文
图6 延迟步数为3,5,d50时第1层的拟合结果
(星为实际值,点为拟合值)
图7 延迟步数为3, 5,d50时第1层的预测结果
(星为实际值,点为拟合值)
共 44 页 第 21 页
┊ ┊ ┊ ┊ ┊ ┊ ┊ ┊ ┊ ┊ ┊ ┊ ┊ 装 ┊ ┊ ┊ ┊ ┊ 订 ┊ ┊ ┊ ┊ ┊ 线 ┊ ┊ ┊ ┊ ┊ ┊ ┊ ┊ ┊ ┊ ┊ ┊ ┊
安徽工业大学 毕业论文
3.当延迟步数为4时的实验结果:
表8给出延迟步数为4时的预测误差指标
表8 延迟步数为4的预测误差指标
最优参数
时间 20100604
a=5 20100607 d=40 20100608 层数=11
20100609
20100610
收盘价 24.8 24.18 24.68 24.89 25.28
预测收盘价 24.1477 24.1665 24.8176 24.5978 25.0028
误差 0.6523 0.0135 -0.1376 0.2922 0.2772
相对误差 2.630% 0.056% 0.558% 1.174% 1.097%
延迟步数为4的拟合曲线和预测曲线由图8和图9给出。
图8 延迟步数为4, 5,d40时第11层的拟合结果
(星为实际值,点为拟合值)
共 44 页 第 22 页
┊ ┊ ┊ ┊ ┊ ┊ ┊ ┊ ┊ ┊ ┊ ┊ ┊ 装 ┊ ┊ ┊ ┊ ┊ 订 ┊ ┊ ┊ ┊ ┊ 线 ┊ ┊ ┊ ┊ ┊ ┊ ┊ ┊ ┊ ┊ ┊ ┊ ┊
安徽工业大学 毕业论文
图9 延迟步数为4,5,d40时第11层的预测结果
(星为实际值,点为拟合值)
4.当延迟步数为5时的实验结果: 表9给出延迟步数为5时的预测误差指标
表9 延迟步数为5的预测误差指标
最优参数
时间 20100604
a=5 20100607 d=80 20100608 层数=20
20100609
20100610
收盘价 24.8 24.18 24.68 24.89 25.28
预测收盘价 24.7026 24.132 24.7638 25.4252 25.0614
误差 0.0974 0.048 -0.0838 -0.5352 0.2186
相对误差 0.393% 0.199% 0.340% 2.150% 0.865%
延迟步数为5的拟合曲线和预测曲线由图10和图11给出。
共 44 页 第 23 页
┊ ┊ ┊ ┊ ┊ ┊ ┊ ┊ ┊ ┊ ┊ ┊ ┊ 装 ┊ ┊ ┊ ┊ ┊ 订 ┊ ┊ ┊ ┊ ┊ 线 ┊ ┊ ┊ ┊ ┊ ┊ ┊ ┊ ┊ ┊ ┊ ┊ ┊
安徽工业大学 毕业论文
图10 延迟步数为5,5、d80,第20层的拟合结果图
(星为实际值,点为拟合值)
图11延迟步数为5,5、d80,第20层的预测结果图
(星为实际值,点为拟合值)
共 44 页 第 24 页
┊ ┊ ┊ ┊ ┊ ┊ ┊ ┊ ┊ ┊ ┊ ┊ ┊ 装 ┊ ┊ ┊ ┊ ┊ 订 ┊ ┊ ┊ ┊ ┊ 线 ┊ ┊ ┊ ┊ ┊ ┊ ┊ ┊ ┊ ┊ ┊ ┊ ┊
安徽工业大学 毕业论文
(三)最优延迟步数的选择
在确定了每一个延迟步数的d的最佳值之后,需要对所挑选出来的各个模型进行横向的比较,从而挑选出逼近能力更强,学习效果更好,预测精度更高的参数模型。下面将根据以上各类预测结果的平均相对误差对预测效果进行评估。见表10
表10 各模型的平均相对预测误差
延迟步数 1 2 3 4 5
x276的预
测误差 0.033% 2.964% 1.593% 2.630% 0.393%
x277的预
测误差 2.747% 1.587% 2.977% 0.056% 0.199%
x278的预
测误差 1.565% 0.287% 0.240% 0.558% 0.340%
x279的预
测误差 0.446% 1.530% 0.491% 1.174% 2.150%
x280的预平均相对
测误差 1.606% 2.855% 1.629% 1.097% 0.865%
1.279% 1.845% 1.386% 1.103% 0.789%
误差大
预测误差 小排序
3 5 4 2 1
观察上表,我们不难看出,当延迟步数为5、5、d80、层数为20时,该多层径向基函数网络对股价的预测达到了很高的精度,预测误差非常小,是一个比较满意的模型。
第五章 总结
本文所建立的多层径向基函数网络模型取得了很好的拟合效果,也具备比较好的短期预测效果。但在试验中发现随着样本延迟步数由小变大的过程,网络的结构和参数也会发生变化。这说明股票价格趋势的波动模式具有短期稳定性与易变性,即模型对样本具有敏感性。利用单一模型进行预测的效果会比较差。金融市场是一个复杂的系统,市场所表现出来的行为实际上是投资者群体行为的一种统计结果。价格趋势的发展过程体现了投资者群体心理特征的变化轨迹,在一个阶段是一种心态,而在另外一个阶段就会变成另外一种心态,正是这种心态的变化导致了价格的变化,进而导致了预测模型的变化。我们进行趋势分析,就是要及时把握群众心理的这种变化,从而预测未来的行情趋势可能向什么方向发展。股票价格预测是一个充满争议的问题,多层径向基网络函数分析方法是对市场行为进行统计分析的有效工具,有着广阔的前景。实证研究表明,股票价格波动具有内在规律性,我们通过对历史价格时间序列数据可以建立价格波动模型,进行短期预测,可以帮助我们发现投资机会、回避投资风险,但在应用过程中,要坚持多方法验证。政策面分析、基本面分析仍然有重要的应用价值,从短期来看,价格与内在价值关系不大,特别对中小投资者,应该充分发挥自身资金量不大,进出市场比较容易的优势,进行波段操作,最大程度地提高投资收益,但从长期来看,价格是由内在价值决定的。本文所建立的时间序列模型,具有较好的短期预测效果,可以实现动态的连续预测,对投资者回避风险和把握买卖时机是有重要实用价值的。
共 44 页 第 25 页
┊ ┊ ┊ ┊ ┊ ┊ ┊ ┊ ┊ ┊ ┊ ┊ ┊ 装 ┊ ┊ ┊ ┊ ┊ 订 ┊ ┊ ┊ ┊ ┊ 线 ┊ ┊ ┊ ┊ ┊ ┊ ┊ ┊ ┊ ┊ ┊ ┊ ┊
安徽工业大学 毕业论文
致谢
首先,我要把我最大的谢意献给我的导师庄健老师。庄老师学问精深,治学严谨,平易近人,自始至终关心、指导和鞭策我的写作,对本文倾注了大量的心血,没有庄老师的无私帮助和悉心指导,我是无论如何完不成本文的。庄老师高尚的人品,无私的奉献精神和严谨的治学态度将使我终生受益。
其次,感谢江海峰老师给我们提供的程序。
在这次论文撰写过程中,不仅收获了知识,也收获了坚毅,更收获了感恩。更让我深刻地明白到,认真、踏实的重要性。衷心感谢参考文献所列的论文作者,他们的工作是我完成本文工作的基础。
最后,谨向评审本论文的所有老师致以最诚挚的谢意,谢谢大家的支持和厚爱。
作者:彭春 2010年6月15日
共 44 页 第 26 页
┊ ┊ ┊ ┊ ┊ ┊ ┊ ┊ ┊ ┊ ┊ ┊ ┊ 装 ┊ ┊ ┊ ┊ ┊ 订 ┊ ┊ ┊ ┊ ┊ 线 ┊ ┊ ┊ ┊ ┊ ┊ ┊ ┊ ┊ ┊ ┊ ┊ ┊
安徽工业大学 毕业论文
参考文献
[1]杨成,程晓玲,殷旅江.基于人工神经网络方法的上市公司股价预测[J]. 统计与决策,2005,12 期(下):106-108. [2]王波,张凤玲.神经网络与时间序列模型在股票预测中的比较[J]. 武汉理工大学学报,2005.12,27(6):69-73.
[3]钟颖,汪秉文.基于遗传算法的BP神经网络时间序列预测模型[J].系统工程与电子技术,2002,24(4):9-11.
[4]林建宇.BP神经网络结合遗传算法进行股市预测的研究[J].福建电脑,2005 年12 期:91-92.
[5]欧阳林群.基于GA-BP的个股超短线预测应用研究[J].微计算机信息,2007,01Z:306-307.
[6]张迎春.基于模糊神经网络的股票价格预测研究[D].南京:南京气象学院,2003. [7]殷洪才,赵春燕,王佳秋.模糊神经网络在股票市场预测中的应用[J].哈尔滨理工大学学报.2007年04 期:85-88.
[8]李建新,胡刚.基于模糊概率的股价波动分析模型[J].系统工程理论与实践,2006 年09 期:33-42.
[9]张红英,吴斌.小波神经网络的研究及其展望[J].西南工学院学报,2002,17(1):8-10,15.
[10]钟满田.基于小波神经网络非线性逼近的股票分析与预测方法研究[D]. 成都:成都理工大学,2007.
[11]廉新宇,吴立锋.基于小波神经网络的股票价格预测[J].商场现代化. 2006482期:331-332.
[12]常松,何建敏.小波包与神经网络相结合的股票价格预测模型[J].东南大学学报(自然科学版),2001.31(5):90-95. [13]王京宝.径向基函数网络(RBF)在股市预测中的应用[J].计算机与信息技术,2007 年第2 期:40-41.
[14]彭英,王艳华.基于RBF网络的股市混沌预测[J].湖南经济管理干部学院学报,2005 年05 期: 72-73
[15]李宗伟,王美娟,郑淑华.基于径向基神经网络的股价预测[J].上海理工大学学报,2002 年 01 期:
[16] Jovina Roman and Akhtar Jameel. Back propagation and Recurrent Neural Networks
共 44 页 第 27 页
┊ ┊ ┊ ┊ ┊ ┊ ┊ ┊ ┊ ┊ ┊ ┊ ┊ 装 ┊ ┊ ┊ ┊ ┊ 订 ┊ ┊ ┊ ┊ ┊ 线 ┊ ┊ ┊ ┊ ┊ ┊ ┊ ┊ ┊ ┊ ┊ ┊ ┊
安徽工业大学 毕业论文
in Financial Analysis of Multiple Stock Market Returns[J].Proceedings of the 29th Annual Hawaii International Conference on System Sciences,1996.
[17]林春燕,朱东华.基于Elman神经网络的股票价格预测研究[J].计算机应用,2006,2(2):446—447转484.
[18]翁苏骏,林熙. BP 网络、RBF 网络和Elman 网络在股价预测中的应用[C], 1999 年中国智能自动化学术会议论文集(上册):205-210. [19]赵佳白.概率神经网络的改进研究及其在股票预测上的应用[D].广州:暨南大学,2007.
[20]武晓炜. 基于人工神经网络的股价预测模型研究[D].大连:大连理工大学,2004. [21] Moody J, Darken C. Fast learning in networks of locally-tuned processing units. Neural Computation, 1989 (1): 281-294
[22] Piggio T, Girosi F, A theory of networks for approximation andlearning. AI Memo No 1140, Artificial Intelligence Laboratory Institute of Technology, Cambridge Mass, 1989
[23] Chen S, Cheng E S, Ai Kadhimi, K. Regularized least squares learning algorithm for constructing radial basis function networks [J], International Journal of Control, 1996,64(5): 829-837
[24] Tyler Holcomb, Manfred Morari. Local lraining for radial basis function networks: toward solving the hidden unit problem. American Control Conference, 1991: 2331-2335
[25] Ho S L, Fei M R, Fu W N, et al. Integrated RBD Network basted estimation strategy of the output characteristics of brushless DC motors[J]. IEEE Transactions on Neural Networks on Magnetics, 2002, 38(2): 1033-1036. [26] Yu D L, Yu D W, Gomm J B, et al. Adaptive RBF model for model-based control[C], Proceedings of the 5 World Congress on Intelligent Control and Automation. IEEE Press, 2004: 78-82.
[27] Li K, Peng J X, Bai E W. A fast nonlinear model identification method [J]. IEEE Transactions on Automatic Control, 2005, 50(80): 1211-1216. [28] Li K, Peng J X, Bai E W. A two-stage algorithm for indentification of nonlinear dynamics system [J] . Automatica, 2006, 42(7): 1189-1197
th
共 44 页 第 28 页
┊ ┊ ┊ ┊ ┊ ┊ ┊ ┊ ┊ ┊ ┊ ┊ ┊ 装 ┊ ┊ ┊ ┊ ┊ 订 ┊ ┊ ┊ ┊ ┊ 线 ┊ ┊ ┊ ┊ ┊ ┊ ┊ ┊ ┊ ┊ ┊ ┊ ┊
安徽工业大学 毕业论文
附录一 程序
延长步数为5程序
Data rawdata;
Input times spj@@; x1=lag(spj); x2=lag2(spj); x3=lag3(spj); x4=lag4(spj); x5=lag5(spj);
if x5=. then delete; t+1;
informat times yymmdd8.; format times yymmdd8.; Cards;
样本数据
;
%macro mxz;
proc transpose data=menx out=cxz prefix=p; data dxz; set cxz;
%do i=1 %to &m ;
if p&i=. or p&i=0 then p&i=mean(of p1-P&m);/* 也可以调整为max */ %end;
proc transpose data=dxz out=menx(keep=mx1-mx5 vx1-vx5); %mend mxz; Run; %macro
ml(cs,n,l,k2,k3,k4,k5,k6,k7,k8,k9,k10,a0,a1,a2,a3,a4,a5,sigs,n1,n2); %do xh=1 %to &cs; %if &xh=1 %then %do; %let m=9; %ml1; %end;
%if &xh ge 2 %then %do; %let m=15;
%let xhh=%eval(&xh-1); %ml2; %end; %end;
proc means data=train&cs min max mean uss range; var abs1-abs&cs ccsq1-ccsq&cs;
output out=trrss(keep=trrss) uss(ccsq&cs)=trrss; proc means data=vvalid&cs min max mean uss range; var abs1-abs&cs ccsq1-ccsq&cs;
output out=varss(keep=varss) uss(ccsq&cs)=varss; proc means data=ttest&cs min max mean uss range; var abs1-abs&cs ccsq1-ccsq&cs;
output out=terss(keep=terss) uss(ccsq&cs)=terss;
%do j=1 %to &cs;
proc gplot data=train&cs;
plot (spj yc&j)*times/overlay;
共 44 页 第 29 页
┊ ┊ ┊ ┊ ┊ ┊ ┊ ┊ ┊ ┊ ┊ ┊ ┊ 装 ┊ ┊ ┊ ┊ ┊ 订 ┊ ┊ ┊ ┊ ┊ 线 ┊ ┊ ┊ ┊ ┊ ┊ ┊ ┊ ┊ ┊ ┊ ┊ ┊
安徽工业大学 毕业论文
symbol1 v=star i=join c=red; symbol2 v=dot i=join c=black; proc gplot data=vvalid&cs;
plot (spj yc&j)*times/overlay; symbol1 v=star i=join c=blue; symbol2 v=dot i=join c=black; run;
proc gplot data=ttest&cs;
plot (spj yc&j)*times/ varis=20 to 30 by 0.25 overlay; symbol1 v=star i=join c=blue; symbol2 v=dot i=join c=black; run;
proc print data=ttest&cs;run; %end;
%mend
ml(cs,n,l,k2,k3,k4,k5,k6,k7,k8,k9,k10,a0,a1,a2,a3,a4,a5,sigs,n1,n2); %macro ml1;
data train valid test; set rawdata;
if t le &n1 then output train;
if t gt &n1 and t le &n2 then output valid; if t gt &n2 then output test;
proc fastclus data=train out=Clust maxclusters=&m noprint; var x1-x5 ;
proc sort data=clust out=flei;by cluster; proc means noprint data=flei; var x1-x5; by cluster;
output out=menx(keep=mx1-mx5 vx1-vx5) mean=mx1-mx5 var=vx1-vx5; %mxz ;
proc transpose data=menx out=ta1&xh(drop=_name_) prefix=m; var mx1-mx5 ;
proc transpose data=menx out=ta2&xh(drop=_name_) prefix=v; var vx1-vx5 ;
data mean1(rename=(m1-m&m=a1-a&m)) mean2(rename=(m1-m&m=b1-b&m)) mean3(rename=(m1-m&m=c1-c&m))
mean4(rename=(m1-m&m=d1-d&m)) mean5(rename=(m1-m&m=e1-e&m)) ; set ta1&xh;
if _n_=1 then output mean1; if _n_=2 then output mean2; if _n_=3 then output mean3; if _n_=4 then output mean4; if _n_=5 then output mean5;
data fc1(rename=(v1-v&m=f1-f&m)) fc2(rename=(v1-v&m=g1-g&m)) fc3(rename=(v1-v&m=h1-h&m))
fc4(rename=(v1-v&m=i1-i&m)) fc5(rename=(v1-v&m=j1-j&m)) ; set ta2&xh;
if _n_=1 then output fc1; if _n_=2 then output fc2; if _n_=3 then output fc3; if _n_=4 then output fc4; if _n_=5 then output fc5; data trexx&xh ;
merge mean1 mean2 mean3 mean4 mean5 fc1 fc2 fc3 fc4 fc5; do j=1 to &n1; output; end;
data vexx&xh ;
merge mean1 mean2 mean3 mean4 mean5 fc1 fc2 fc3 fc4 fc5;
共 44 页 第 30 页
┊ ┊ ┊ ┊ ┊ ┊ ┊ ┊ ┊ ┊ ┊ ┊ ┊ 装 ┊ ┊ ┊ ┊ ┊ 订 ┊ ┊ ┊ ┊ ┊ 线 ┊ ┊ ┊ ┊ ┊ ┊ ┊ ┊ ┊ ┊ ┊ ┊ ┊
安徽工业大学 毕业论文
do j=1 to &n2-&n1; output; end;
data texx&xh ;
merge mean1 mean2 mean3 mean4 mean5 fc1 fc2 fc3 fc4 fc5; do j=1 to &n-&n2; output; end;
data hb&xh(keep=x1-x5 spj ex1-ex&m times); merge train trexx&xh; %do i=1 %to &m;
x1&i=(x1-a&i)**2/(&l*f&i); x2&i=(x2-b&i)**2/(&l*g&i); x3&i=(x3-c&i)**2/(&l*h&i); x4&i=(x4-d&i)**2/(&l*i&i); x5&i=(x5-e&i)**2/(&l*j&i);
xhb&i=x1&i+x2&i+x3&i+x4&i+x5&i; ex&i=exp(-0.5*xhb&i); %end;
proc reg data=hb&xh outest=est&xh(keep=ex1-ex&m); model spj=ex1-ex&m/noint;
output out=b&xh(drop=ex1-ex&m) p=py&xh r=ry;
data vplot&xh(rename=(ex1-ex&m=p1-p&m)); set est&xh; do nn=1 to &n2-&n1;output; end;
data tplot&xh(rename=(ex1-ex&m=p1-p&m)); set est&xh; do nn=1 to &n-&n2;output; end;
data valid&xh;py&xh=0;
merge valid vexx&xh vplot&xh; %do i=1 %to &m;
x1&i=(x1-a&i)**2/(&l*f&i); x2&i=(x2-b&i)**2/(&l*g&i); x3&i=(x3-c&i)**2/(&l*h&i); x4&i=(x4-d&i)**2/(&l*i&i); x5&i=(x5-e&i)**2/(&l*j&i);
xhb&i=x1&i+x2&i+x3&i+x4&i+x5&i; ex&i=exp(-0.5*xhb&i); he&i=p&i*ex&i; py&xh+he&i; %end;
ccc&xh=spj-py&xh;
keep x1-x5 ccc&xh py&xh spj times;
data test&xh;py&xh=0;
merge test texx&xh tplot&xh; %do i=1 %to &m;
x1&i=(x1-a&i)**2/(&l*f&i); x2&i=(x2-b&i)**2/(&l*g&i); x3&i=(x3-c&i)**2/(&l*h&i); x4&i=(x4-d&i)**2/(&l*i&i); x5&i=(x5-e&i)**2/(&l*j&i);
xhb&i=x1&i+x2&i+x3&i+x4&i+x5&i; ex&i=exp(-0.5*xhb&i); he&i=p&i*ex&i; py&xh+he&i; %end;
共 44 页 第 31 页
┊ ┊ ┊ ┊ ┊ ┊ ┊ ┊ ┊ ┊ ┊ ┊ ┊ 装 ┊ ┊ ┊ ┊ ┊ 订 ┊ ┊ ┊ ┊ ┊ 线 ┊ ┊ ┊ ┊ ┊ ┊ ┊ ┊ ┊ ┊ ┊ ┊ ┊
安徽工业大学 毕业论文
ccc&xh=spj-py&xh;
keep spj x1-x5 ccc&xh py&xh times;
data a ; set b&xh; RY2=RY; run;
Data train&xh; Set b&xh; Yc&xh=py&xh;
Cc&xh=spj-Yc&xh; Abs&xh=abs(cc&xh); ccsq&xh=cc&xh**2;
Data vvalid&xh; Set valid&xh; Yc&xh=py&xh; Cc&xh=ccc&xh;
Abs&xh=abs(cc&xh); ccsq&xh=cc&xh**2;
Data ttest&xh; Set test&xh; Yc&xh=py&xh; Cc&xh=ccc&xh;
Abs&xh=abs(cc&xh); ccsq&xh=cc&xh**2;
%mend ml1; %macro ml2;
proc fastclus data=a out=Clust maxclusters=&m noprint; var x1-x5 ry2;
proc sort data=clust out=flei;by cluster; proc means noprint data=flei; var x1-x5; by cluster;
output out=menx(keep=mx1-mx5 vx1-vx5) mean=mx1-mx5 var=vx1-vx5; %mxz ;
proc transpose data=menx out=ta1&xh(drop=_name_) prefix=m; var mx1-mx5;
proc transpose data=menx out=ta2&xh(drop=_name_) prefix=v; var vx1-vx5;
data mean1(rename=(m1-m&m=a1-a&m))
mean2(rename=(m1-m&m=b1-b&m))mean3(rename=(m1-m&m=c1-c&m))
mean4(rename=(m1-m&m=d1-d&m)) mean5(rename=(m1-m&m=e1-e&m)) ; set ta1&xh;
if _n_=1 then output mean1; if _n_=2 then output mean2; if _n_=3 then output mean3; if _n_=4 then output mean4; if _n_=5 then output mean5;
data fc1(rename=(v1-v&m=f1-f&m)) fc2(rename=(v1-v&m=g1-g&m)) fc3(rename=(v1-v&m=h1-h&m))
fc4(rename=(v1-v&m=i1-i&m)) fc5(rename=(v1-v&m=j1-j&m)); set ta2&xh;
if _n_=1 then output fc1; if _n_=2 then output fc2; if _n_=3 then output fc3; if _n_=4 then output fc4;
共 44 页 第 32 页
┊ ┊ ┊ ┊ ┊ ┊ ┊ ┊ ┊ ┊ ┊ ┊ ┊ 装 ┊ ┊ ┊ ┊ ┊ 订 ┊ ┊ ┊ ┊ ┊ 线 ┊ ┊ ┊ ┊ ┊ ┊ ┊ ┊ ┊ ┊ ┊ ┊ ┊
安徽工业大学 毕业论文
if _n_=5 then output fc5; data trexx&xh ;
merge mean1 mean2 mean3 mean4 mean5 fc1 fc2 fc3 fc4 fc5; do j=1 to &n1; output; end;
data vexx&xh ;
merge mean1 mean2 mean3 mean4 mean5 fc1 fc2 fc3 fc4 fc5; do j=1 to &n2-&n1; output; end;
data texx&xh ;
merge mean1 mean2 mean3 mean4 mean5 fc1 fc2 fc3 fc4 fc5; do j=1 to &n-&n2; output; end;
data hb&xh(keep=x1-x5 ry spj ex1-ex&m); merge a trexx&xh; %do i=1 %to &m;
x1&i=(x1-a&i)**2/(&l*f&i); x2&i=(x2-b&i)**2/(&l*g&i); x3&i=(x3-c&i)**2/(&l*h&i); x4&i=(x4-d&i)**2/(&l*i&i); x5&i=(x5-e&i)**2/(&l*j&i);
xhb&i=x1&i+x2&i+x3&i+x4&i+x5&i; ex&i=exp(-0.5*xhb&i); %end;
proc reg data=hb&xh outest=est&xh(keep=ex1-ex&m); model ry=ex1-ex&m/noint;
output out=b&xh(drop=ex1-ex&m) p=py&xh r=ry1;
data vplot&xh(rename=(ex1-ex&m=p1-p&m)); set est&xh; do nn=1 to &n2-&n1;output; end;
data tplot&xh(rename=(ex1-ex&m=p1-p&m)); set est&xh; do nn=1 to &n-&n2;output; end;
data valid&xh;py&xh=0;
merge valid&xhh vexx&xh vplot&xh; %do i=1 %to &m;
x1&i=(x1-a&i)**2/(&l*f&i); x2&i=(x2-b&i)**2/(&l*g&i); x3&i=(x3-c&i)**2/(&l*h&i); x4&i=(x4-d&i)**2/(&l*i&i); x5&i=(x5-e&i)**2/(&l*j&i);
xhb&i=x1&i+x2&i+x3&i+x4&i+x5&i; ex&i=exp(-0.5*xhb&i); he&i=p&i*ex&i; py&xh+he&i; %end;
ccc&xh=ccc&xhh-py&xh;
keep spj x1-x5 ccc&xh py&xh ;
data test&xh;py&xh=0;
merge test&xhh texx&xh tplot&xh; %do i=1 %to &m;
共 44 页 第 33 页
┊ ┊ ┊ ┊ ┊ ┊ ┊ ┊ ┊ ┊ ┊ ┊ ┊ 装 ┊ ┊ ┊ ┊ ┊ 订 ┊ ┊ ┊ ┊ ┊ 线 ┊ ┊ ┊ ┊ ┊ ┊ ┊ ┊ ┊ ┊ ┊ ┊ ┊
安徽工业大学 毕业论文
x1&i=(x1-a&i)**2/(&l*f&i); x2&i=(x2-b&i)**2/(&l*g&i); x3&i=(x3-c&i)**2/(&l*h&i); x4&i=(x4-d&i)**2/(&l*i&i); x5&i=(x5-e&i)**2/(&l*j&i);
xhb&i=x1&i+x2&i+x3&i+x4&i+x5&i; ex&i=exp(-0.5*xhb&i); he&i=p&i*ex&i; py&xh+he&i; %end;
ccc&xh=ccc&xhh-py&xh;
keep spj x1-x5 ccc&xh py&xh ;
data c; set b&xh; drop ry;
data a(rename=(ry1=ry)); set c;
RY2=RY1*&&k&xh;
data train&xh(rename=(ry1=ry&xh)); merge train&xhh b&xh;
yc&xh=py&xh+yc&xhh; cc&xh=spj-yc&xh; abs&xh=abs(cc&xh); ccsq&xh=cc&xh**2;
data vvalid&xh;
merge vvalid&xhh valid&xh; yc&xh=py&xh+yc&xhh; cc&xh=spj-yc&xh; abs&xh=abs(cc&xh); ccsq&xh=cc&xh**2; run;
data ttest&xh;
merge ttest&xhh test&xh; yc&xh=py&xh+yc&xhh; cc&xh=spj-yc&xh; abs&xh=abs(cc&xh); ccsq&xh=cc&xh**2; run;
%mend ml2;
%ml(20,276,80,5,5,5,5,5,5,5,5,5,0.8,0.1,0.4,0.2,0.7,0.8,1,270,270); run;
共 44 页 第 34 页
┊ ┊ ┊ ┊ ┊ ┊ ┊ ┊ ┊ ┊ ┊ ┊ ┊ 装 ┊ ┊ ┊ ┊ ┊ 订 ┊ ┊ ┊ ┊ ┊ 线 ┊ ┊ ┊ ┊ ┊ ┊ ┊ ┊ ┊ ┊ ┊ ┊ ┊
安徽工业大学 毕业论文
附录二 外文文献
Neural Networks in Sensor Signal Processing
The basic principle
Neural network is a system do not need to select the basis function method for nonlinear function approximation. This paper describes the highly nonlinear neural network capacity, sensor signal processing to achieve. As the neural network is based on the object's input 2 output, continuous learning of network parameters to achieve the output parameters from the input parameters to the nonlinear mapping; also available from the mechanism according to the object model and the actual operation of new data samples adaptive learning, especially through continuous real-time learning, can be slow to adapt to changes in object parameters. Therefore, this method can overcome the difficulties inherent in modeling the mechanism. Neural networks can do a large amount of information processing tasks, the application involves a wide range of areas. To sum up, neural network information processing tasks include:
(1) mathematical mapping approximation. Development of a suitable function f: A → B -organized way to respond to the following sample sets: (x 1, y 1), (x 2, y 2), ⋯, (x l, y l) (where y i = F (x i) or y i = F (x i) + n 0, (where n 0 is the noise)). Calculated as the identification and classification can be so abstract mathematical mapping of a similar.
(2) associative memory. Is the perfect realization mode and restore the mutual memories and other related models. Neural networks store the connection information distributed weights, the network has high fault-tolerance and robustness, while the pattern recognition there is often noise or partial loss of input mode, this feature of the network enabling them to for pattern recognition.
The neural network applied to the sensor signal processing involves two important issues: model preprocessing transform and pattern recognition. Preprocessing transformation is the acceptance of a formal model by applying neural networks to convert it to more forms of pattern you want to or can; and pattern recognition is to map a model to other types or categories of operations. Shows that neural network applications in sensor signal processing is based on neural network function approximation capability, and use this ability to model the sensor. There is much literature on the function approximation ability of neural networks have been studied, and the sensor system based on neural network modeling of a direct inverse modeling method, is 2 inverse modeling, reverse 2 inverse modeling method.
Direct inverse modeling method is: use a different setting signal u d input to the unknown sensor, measuring the corresponding output y, for u d And y to train a neural network to the network to unknown sensor output y as input, the network's output exactly equals u unknown sensor input u d . At this time the error u 2 u d Can be used as network training.
Are two inverse modeling method is: in the unknown dynamic system sensor (positive) model based on inverse dynamics model. There are three different learning methods: ① sensor 2 inverse learning. The direct use of neural network inverse system set to the expectations of the real input and unknown sensor _ the difference between the international input to adjust the network weights. This method is based on the overall
共 44 页 第 35 页
┊ ┊ ┊ ┊ ┊ ┊ ┊ ┊ ┊ ┊ ┊ ┊ ┊ 装 ┊ ┊ ┊ ┊ ┊ 订 ┊ ┊ ┊ ┊ ┊ 线 ┊ ┊ ┊ ┊ ┊ ┊ ┊ ┊ ┊ ┊ ┊ ┊ ┊
安徽工业大学 毕业论文
error of the right to adjust the value of the network, you must know the sensor model. ② Model 2 inverse system is learning. First, a neural network built with sensor (unknown) model. Set to the inverse system using neural networks and sensors are expected input neural network model to adjust the difference between the output of neural network inverse system weight. Drawback is that the accuracy of inverse system model directly with the newly established model accuracy is related to. ③ 2 sensor 2 is inverse model learning. This method avoids the shortcomings of ②, ① Zeyi based learning framework. That is directly set to the inverse model of the expected input and output of the sensor network, the difference between the adjusted weights.
Inverse Modeling Method 2 is the inverse: the inverse system model and the two unknown sensor circuit together constitute learning. There are channels in the neural network controller, which controls the sensor network so that the input is complex. Of course, this method requires prior known inverse dynamics model of the sensor.
Application examples
1. Nonlinear correction
Measurement Technology of the Non-electricity is widely used with various types of sensors, they used to charge non-electricity into. Most of the sensor output characteristic is nonlinear, due to its conversion principle is nonlinear. In order for the entire measurement range sensor sensitivity is a constant, that is, the input 2 sensor output characteristic is a straight line, in the traditional analog indicating meters are often used in three ways: 1) reduce the measuring range, taking approximation; 2) non- scale linear direction; 3) nonlinear correction link. But with the expanded measurement range, measurement accuracy is ever increasing, these methods may lose their value. For example, the external rotation-type concentration sensor input 2 output characteristics of the obvious non-linear, you can use neural network correction of its characteristics. Figure 1 is a nonlinear correction using neural network principles.
Suppose sensor output characteristics of nonlinear input 2 y = f (x), in order to achieve non-linear error correction, allows the sensor output y through calibration using neural network. Take the characteristics of the x est link = F (y), if so F (y) = f 21 (y), then there is x est = F 21 (y) = x. So long as that feature f 21 can eliminate the nonlinear sensor. Specific methods are: the concentration sensor output as input of neural network y, and will be tested concentration x as a neural network output, the neural network training (learning iteration method with neural network weights correction) until the neural network output value reached the mean square estimation error is small enough, the learning process ended. Then neural network can be used to correct for nonlinear prediction, the output shall be corrected results x est. PH value of this method in optical fiber sensors, color sensors and nonlinear inductance sensor error correction also has been applied.
2. Self-test and fault diagnosis
When the production process monitoring, control and optimization which are based on certain measurements of process variables such as temperature, flow, concentration-based. Therefore, the data obtained from the measured process variable reliable, accurate, consistent estimates of the production process is essential. Sensor fault error and failure to make the system run performance degradation or lead to system collapse. To solve this problem, people made a variety of data checking and gross error detection. Most of these methods is the statistical theory and optimization methods are combined, computing capacity, long time, it is difficult to online applications. For gross error detection, the
共 44 页 第 36 页
┊ ┊ ┊ ┊ ┊ ┊ ┊ ┊ ┊ ┊ ┊ ┊ ┊ 装 ┊ ┊ ┊ ┊ ┊ 订 ┊ ┊ ┊ ┊ ┊ 线 ┊ ┊ ┊ ┊ ┊ ┊ ┊ ┊ ┊ ┊ ┊ ┊ ┊
安徽工业大学 毕业论文
deviation vector r = [r i] T values will provide some useful information, so you can bias vector r as the neural network input, the output of the neural network corresponds to the measured variables. Specific methods are: pretreatment of the deviation vectors, which use the r i In addition to its standard deviation of Vi, the numerical S i = R i / V i As neural network input; network output, as measured variables do not exist in the time taken to be 011 gross errors, when there is gross error in the measured variables when taken as 019, to train the network. Trained network can be used for gross error detection.
Figure 2 is based on neural network theory of single sensor fault detection. The method is characterized by: using a single sensor's output signal is a neural network prediction model, and then use prediction models to forecast the sensor output and actual output sensor to judge whether the difference between failure. Neural Network Predictor for sensor output, the first m-value x (k), x (k + 1), ⋯, x (k + m - 1) predicted the first m + 1 times the value of ^ x (k + m) , be the first m + 1 and sensor time difference between the actual output of a detection threshold and compared to determine whether the failure of this sensor.
Figure 2, a single sensor based on neural network fault detection 3. Smart structure sensor array signal processing
Imitation of life will have the function of material embedded in the matrix material, so that the components made with the expectation of intelligent features, such a structure known as smart materials and structures. Elements of intelligent structures currently focused on the application of sensor technology on the structure of some of the state parameters were measured. With its small size optical sensor, low loss, high sensitivity, and resistance to electromagnetic interference, electrical insulation and good, wide bandwidth, can "sense" can "Biography", easy to implement distributed and the array of sensor network, which is The most promising sensor technology for smart structures. Figure 3 (a) is a method of using optical fiber made of criss-cross arrangement of optical fiber microbending sensor arrays in the intelligent component of the arrangement. Can be achieved using the array of monitoring and locating structural damage. Figure 3 (b) the use of neural network processing sensor array output data, and determine the strength and location of mechanical damage block diagram.
4. Filtering and denoising
In addition to noise filtering and sensor information processing is the basic operation. In the adaptive noise canceling (ANC), the entire ANC system is divided into two channels: the main channel and reference channel. When the reference noise is a nonlinear transform of the primary noise, if the linear transformation of linear adaptive algorithm to approximate nonlinear transformation, in order to achieve sufficiently high accuracy, the weight coefficient vector used to estimate the dimension of the explosive increase of the estimated speed of adaptive filter must be greatly reduced, their performance dropped substantially, it is difficult to achieve the purpose of real-time applications, and you should use nonlinear ANC. As the radial basis function (RBF) network has the good performance of the nonlinear function approximation, which can be used RBF network based nonlinear ANC.
Figure 4 Neural network model of weak signal extraction
In target tracking, multiple target detection and other engineering fields, are related to the strong background noise from the detection of a weak signal problem. Based on BP neural network structure and algorithm methods can be extracted from the broadband background noise weak useful signal. This method is the extraction of weak signals in
共 44 页 第 37 页
┊ ┊ ┊ ┊ ┊ ┊ ┊ ┊ ┊ ┊ ┊ ┊ ┊ 装 ┊ ┊ ┊ ┊ ┊ 订 ┊ ┊ ┊ ┊ ┊ 线 ┊ ┊ ┊ ┊ ┊ ┊ ┊ ┊ ┊ ┊ ┊ ┊ ┊
安徽工业大学 毕业论文
the network node connection weights vector domain, and therefore a fundamental solution to extract the signal of the frequency of choice. BP network weight vector method shown in Figure 4. Detection system for the broadband noise input x k And to extract the weak signal S k Superposition. Taking the expected value of m x background noise As expected response d k, ie d k = E (x k) = m x assume the background noise is stationary, then m x Available time average approximation. Suppose before the appearance of weak signals, the network learning process is over, that is, the expected value of the network connection weight matrix converges to the background noise has been determined by the best weight vector W 3 x. Then there are the right offset vector
V k = W k - W 3 x the formula, V k To offset the weight, W k For the weak signal containing background noise and weight vector. When before the appearance of weak signals, V k swim around in the zero vector, a weak signal appeared to change the input characteristics to produce new optimal weight vector W 3 x 1 BP algorithm makes the weight vector Wk and W 3 x as much as possible to match , so E (V k) is not zero vectors. Methods BP network weight vector E (V k) and each component of the decision as a function of weak signal detection.
5. Compensation for environmental factors
Optical fiber current sensor based on the medium to Faraday (Faraday) current magneto-optical effect based sensors. With the traditional electromagnetic current transformer than in high-voltage high-current measurement applications using optical technology has obvious advantages: to meet the insulation requirements, no magnetic saturation, anti-electromagnetic interference, wide frequency response, ease of remote sensing and telemetry, compact and light weight. But truly market-based optical current sensors are still rare, and the fundamental reason is that environmental factors of optical linear birefringence effect on the measurement results. Literature of the three-phase optical current sensor Birefringence; literature were proposed based on BP and RBF neural network linear birefringence effect optical current sensor compensation method (Figure 5). The optical current sensor's output as input of neural network signal, the true value of the measured current (obtained by means of other measurements can be used) as a neural network output signal, input 2-output relationship in this sample set formed by the neural network training. Trained network can be used to compensate the linear birefringence effect of the forecast. The results showed that: the network prediction and experimental values with good consistency.
Figure 5 Neural network linear birefringence effect optical current sensor compensation 6. Multi-sensor information fusion
Data fusion is to come from multi-sensor data and information, according to established rules, combined with a comprehensive analysis of intelligence, and on this basis, the demand for the users of information, such as decision-making tasks. In short, the basic purpose of data fusion by combining, you can input data than from any single element to get more information. There are usually cross-sensitivity sensor, the output value of the performance of the sensor not only depends on a constant. When other parameters change, the output value should change. For example: a pressure sensor, when the pressure P constant and the temperature T changes, the output value of U is also changed. Then the pressure sensor on the existence of cross sensitivity to temperature T. The existence of cross sensitivity of sensors, the performance of unstable, low accuracy. Multi-sensor information fusion technology is through the detection of multiple parameters and use certain information processing methods (here using neural network technology, see Figure (6) to improve the accuracy of each parameter's purpose.
共 44 页 第 38 页
┊ ┊ ┊ ┊ ┊ ┊ ┊ ┊ ┊ ┊ ┊ ┊ ┊ 装 ┊ ┊ ┊ ┊ ┊ 订 ┊ ┊ ┊ ┊ ┊ 线 ┊ ┊ ┊ ┊ ┊ ┊ ┊ ┊ ┊ ┊ ┊ ┊ ┊
安徽工业大学 毕业论文
Figure 6, the sensor based on neural network information fusion
Conclusion
(1) neural network with the expression of knowledge and information distributed, large-scale parallel processing, automatic acquisition, knowledge processing adaptability, fault tolerance and associative memory and so on. Sensor information is generally difficult to identify with the model, the strong nonlinear characteristics, to be measured accurately be tremendous difficulties. Neural network just to meet these characteristics, you can input mode to output mode complete the complex mapping. Therefore, the combination of sensor and neural network technology development is inevitable. This article provides the neural network for sensor signal processing theory and methods for improving the sensor performance, and tap the potential of the sensor plays an important role.
(2) need to be clear is that in engineering practice will be met with some more difficult to detect with sensing techniques can not even detect the process variable, then, based on neural network soft sensor technology is an effective way to solve the problem. It is based on the production process and the process of association between variables in order to use the sensor technology that can detect the process variables and the corresponding mathematical model, estimated by the neural network difficult to detect as a "sensing" technology.
神经网络在传感信号处理中的应用
一、基本原理
神经网络是一种不需要选取基函数系的非线性函数逼近方法。本文利用神经网络的高度非线性描述能力, 实现传感信号的处理。由于神经网络是根据对象的输入2输出信息, 不断地对网络参数进行学习, 以实现从输入参数到输出参数的非线性映射; 还可以根据来自机理模型和实际运行对象的新数据样本进行自适应学习, 尤其是通过不断的实时学习, 可以适应对象参数的缓慢变化。因此, 这种方法克服了机理建模所存在的困难。神经网络可以完成大量的信息处理任务, 其应用涉及相当广泛的领域。归纳起来, 神经网络的信息处理任务主要包括:
(1) 数学上的映射逼近。开发一种合适的函数f :A < Rn →B < Rn ,以自组织的方式响应以下的样本集:( x1 , y1) , ( x2 , y2) , ⋯ , ( xl , yl) (这里yi = f ( xi) 或yi =f ( xi) + n0 , (其中n0 为噪声) ) 。象识别和分类等计算都可以抽象成这样的一种近似的数学映射。
(2) 联想记忆。是指实现模式完善、恢复相关模式的相互回忆等。神经网络的信息分布式存储于连接权系数中, 使网络具有很高的容错性和鲁棒性, 而模式识别中往往存在噪声干扰或输入模式的部分损失,网络的这一特点使其成功地用于模式识别问
共 44 页 第 39 页
┊ ┊ ┊ ┊ ┊ ┊ ┊ ┊ ┊ ┊ ┊ ┊ ┊ 装 ┊ ┊ ┊ ┊ ┊ 订 ┊ ┊ ┊ ┊ ┊ 线 ┊ ┊ ┊ ┊ ┊ ┊ ┊ ┊ ┊ ┊ ┊ ┊ ┊
安徽工业大学 毕业论文
题。
将神经网络应用到传感信号处理涉及到两个重要的问题: 模式预处理变换和模式识别。预处理变换是指接受一种形式模式, 应用神经网络把它转换为更多想要或可用形式的模式; 而模式识别则是把一模式映射到其它类型或类别的操作。可见, 神经网络在传感信号处理应用的基础是神经网络的函数逼近能力, 并利用这一能力对传感器进行建模。已有许多文献对神经网络的函数逼近能力进行了研究, 而基于神经网络的传感系统的建模有直接逆系统建模法、正2逆系统建模法、逆2逆系统建模法。
直接逆系统建模法是指:采用不同的设定信号ud输入给未知传感器,测量其相应的输出y ,以ud 和y来训练一个神经网络,使这个网络把未知传感器的输出y 作为网络输入时,网络的输出u 正好等于未知传感器的输入ud 。这时误差u2ud 可作为网络的训练。
正2逆系统建模法是指: 在未知传感器的动力学系统(正) 模型的基础上建立逆动力学模型。有三种不同学习法: ①传感器2逆系统学习法。即直接利用设定给逆系统神经网络的期望输入和未知传感器的实_际输入之差来调节网络的权重。这种方法在整体误差的基础上调节网络权值时, 必须知道传感器的模型。②正模型2逆系统学习法。首先用一个神经网络建立起传感器的(未知的) 数学模型。利用设定给逆系统神经网络的期望输入和传感器正的神经网络模型的输出之差来调节逆系统神经网络的权值。缺点是逆系统模型的准确度直接与新建立的正模型精度有关。③传感器2正模型2逆系统学习法。这种方法避免了②的缺点, 学习框架则以①为基础。即直接利用设定给逆模型的期望输入和传感器的输出之差调节网络的权值。
逆2逆系统建模法是指: 由两个逆系统模型和未知传感器一起构成学习回路。在正通道上有神经网络控制器, 它控制传感器使之复现网络的输入。当然该方法需要事先已知传感器的逆动力学模型。 二、应用举例 1.非线性校正
在非电量电测技术中广泛使用着各类传感器, 他们用来将非电量转化为电量。大多数传感器的输出特性是非线性的, 这是由于其转换原理为非线性的。为了使传感器在整个测量范围内的灵敏度是一个常数,即传感器的输入2输出特性是一条直线, 在
共 44 页 第 40 页
┊ ┊ ┊ ┊ ┊ ┊ ┊ ┊ ┊ ┊ ┊ ┊ ┊ 装 ┊ ┊ ┊ ┊ ┊ 订 ┊ ┊ ┊ ┊ ┊ 线 ┊ ┊ ┊ ┊ ┊ ┊ ┊ ┊ ┊ ┊ ┊ ┊ ┊
安徽工业大学 毕业论文
传统的模拟指示仪表中常采用三种方法: 1) 缩小测量范围,取近似值; 2) 采用非线性指示刻度; 3) 加入非线性校正环节。但随着测量范围不断扩大, 对测量准确度的要求不断提高, 上述这些方法可能失去其使用价值。例如, 外旋转式浓度传感器的输入2输出特性存在着明显的非线性, 可以用神经网络实现其特性的校正。图1 是用神经网络实现非线性校正的原理。
假设传感器具有非线性输入2输出特性y = f( x) , 为了实现非线性误差的校正, 可使传感器的输出y 通过校正用神经网络。取该环节的特性为x =F ( y ) , 如果令F ( y ) = f21 ( y ) , 则有xest = f21( y) = x 。所以只要知道特性f21 即可消除传感器的非线性。具体做法是: 将浓度传感器的输出视为神经网络的输入y , 而将待测浓度值x 作为神经网络的输出, 对神经网络进行训练(用学习迭代方法对神经网络的权值进行修正) , 直至神经网络输出值的估计误差均方值达到足够小, 学习过程才告结束。这时神经网络就可用来作非线性校正预测, 其输出即为校正结果xest 。这种方法在光纤PH 值传感器、色度传感器和电感测微仪的非线性误差校正中也已获得应用。
2.自检验与故障诊断
当对生产过程进行监测、控制以及优化时, 都是以过程变量的某些测量值如温度、流量、浓度等为依据的。因此从测量数据中获得过程变量可靠、精确、一致的估计值对生产过程至关重要。传感器的过失误差和故障可使系统运行性能下降或导致系统瘫痪。针对这一问题, 人们提出了多种数据校核及过失误差检测方法。这些方法大多是统计理论和最优化方法的结合, 计算量大、时间长, 因此难以在线应用。对于过失误差检测而言, 偏差向量r = [ ri ] T 的数值将提供一些有用的信息, 因此可以将偏差向量r作为神经网络的输入, 神经网络的输出则对应于各测量变量。具体做法是: 将偏差向量进行预处理, 即用各ri 除以其标准差Vi , 所得数值Si = ri/ Vi 作为神经网络的输入; 网络的输出, 当测量变量中不存在过失误差时取为011 , 当测量变量中存在过失误差时取为019 , 对网络进行训练。训练后的网络即可用来进行过失误差的检测。
图2 是基于神经网络的单传感器故障检测原理。该方法的特点是: 利用单传感器的输出信号建立神经网络预测模型, 然后利用预测模型对传感器的预测输出和实际输出
est
共 44 页 第 41 页
┊ ┊ ┊ ┊ ┊ ┊ ┊ ┊ ┊ ┊ ┊ ┊ ┊ 装 ┊ ┊ ┊ ┊ ┊ 订 ┊ ┊ ┊ ┊ ┊ 线 ┊ ┊ ┊ ┊ ┊ ┊ ┊ ┊ ┊ ┊ ┊ ┊ ┊
安徽工业大学 毕业论文
之差判断传感器是否发生故障。神经网络预测器根据传感器输出的前m 个值x ( k) ,x
( k + 1) , ⋯, x ( k + m - 1) 预测得到第m + 1 时刻的值^x ( k + m) , 将其与传感器第m + 1 时刻的实际输出之差与某一检测阈值相比较, 判断此传感器是否发生故障。
3 .智能结构中传感阵列的信号处理
将具有仿生命功能的材料融合于基体材料中, 使制成的构件具有人们期望的智能功能, 这种结构称为智能材料结构。目前智能结构的元件主要集中于应用传感技术对结构中的一些状态参数进行测量。光纤传感器由于具有体积小、损耗低、灵敏度高, 并且抗电磁干扰、电绝缘性好、带宽大、既可“感”又可“传”, 易于实现分布式和阵列化传感网络, 因而是最有希望用于智能结构的传感技术。采用光纤纵横交错排列的方法制成的光纤微弯传感阵列在智能化构件中的安排。利用该阵列可以实现结构损伤的监测和定位。利用神经网络处理传感阵列的输出数据, 并确定机械损伤强度和位置的原理框图。
4.滤波与除噪
滤波与除噪是传感信息处理中的基本操作。在自适应除噪(ANC) 中, 整个ANC 系统分为两个通道:主通道与参考通道。当参考通道中噪声是主通道噪声的非线性变换时, 如果线性自适应算法用线性变换逼近非线性变换, 为了达到足够高的精度, 用于估计的权系数向量的维数将爆炸性增大, 自适应滤波器的估计速度必然大为降低, 其性能大幅度下降, 难以达到实时应用的目的, 此时应采用非线性ANC。由于径向基函数(RBF) 网络具有良好的非线性函数逼近性能, 因而可以采用基于RBF 网络的非线性ANC 。
在目标跟踪、多目标检测等工程领域, 都涉及到从强的背景噪声中提取弱信号的问题。基于BP 神经网络结构及算法的方法, 可从宽带背景噪声中提取微弱有用信号。该方法对微弱信号的提取是在网络节点连接权向量域进行的, 因此从根本上解决了对提取信号的频率选择问题。BP 网络权向量方法如图4所示。检测系统的输入为宽带噪声xk 和要提取的微弱信号Sk 的叠加。取背景噪声的期望值mx 作为期待响应dk , 即
dk = E( xk) = mx假设背景噪声是平稳的, 则mx 可用时间平均值近似估计。假设弱信
共 44 页 第 42 页
┊ ┊ ┊ ┊ ┊ ┊ ┊ ┊ ┊ ┊ ┊ ┊ ┊ 装 ┊ ┊ ┊ ┊ ┊ 订 ┊ ┊ ┊ ┊ ┊ 线 ┊ ┊ ┊ ┊ ┊ ┊ ┊ ┊ ┊ ┊ ┊ ┊ ┊
安徽工业大学 毕业论文
号出现之前, 网络学习过程已经结束, 即网络连接权矩阵的期望值已收敛于由背景噪声所确定的最佳权向量W 3x。于是有偏移权向量
Vk = Wk - W 3x式中, Vk 为权值偏移量, Wk 为含有背景噪声和弱信号的权向量。当弱信号出现之前, Vk 在零向量周围游动, 弱信号的出现改变了输入特性, 从而产生新的最佳权向量W 3x 1B P 算法使权向量Wk 尽可能与W 3x相匹配, 这样E ( Vk) 则不为零向量。B P 网络权向量方法取E ( Vk) 各分量之和作为检测弱信号的判决函数。 5.环境影响因素的补偿
光学电流传感器是以光纤为介质, 以法拉第(Faraday) 磁光效应为基础的电流传感元件。与传统的电磁式电流互感器相比, 在高电压大电流测量的应用中采用光学技术具有明显的优越性: 满足绝缘要求、无磁饱和现象、抗电磁干扰、响应频域宽、便于遥感和遥测、结构紧凑、重量轻等。但目前真正可立足于市场的光学电流传感器产品仍是凤毛麟角, 其根本原因在于环境因素引起的光纤线性双折射效应对测量结果的影响。文献研究了三相光学电流传感器的双折射效应; 文献分别提出了基于BP和RBF 神经网络的光学电流传感器线性双折射效应的补偿方法。将光学电流感器的输出作为神经网络的输入信号, 将被测电流的真实值(可用其他测量手段获得) 作为神经网络的输出信号, 用这种输入2输出关系形成的样本集对神经网络进行训练。经过训练的网络就可以用来进行线性双折射效应补偿的预测。结果表明: 网络的预测值与实验值具有很好的一致性。 6.多传感器信息融合
数据融合是把来自多传感器的数据和信息, 根据既定的规则, 分析结合为一个全面的情报, 并在此基础上为用户提供需求的信息, 诸如决策、任务等。简单地说, 数据融合的基本目的就是通过组合, 可以比从任何单个输入数据元素获得更多的信息。通常传感器都存在交叉灵敏度, 表现在传感器的输出值不只决定于一个常量。当其它参量变化时输出值也要发生变化。例如: 一个压力传感器, 当压力P恒定而温度T 变化时, 其输出值U 也发生改变。那么这个压力传感器就存在有对温度T 的交叉灵敏度。存在交叉灵敏度的传感器, 其性能不稳定, 测量精度低。多传感器信息融合技术就是通过对多个参数的检测并采用一定的信息处理方法(这里采用神经网络技术, 达到提高每一个参量测量精度的目的。
共 44 页 第 43 页
┊ ┊ ┊ ┊ ┊ ┊ ┊ ┊ ┊ ┊ ┊ ┊ ┊ 装 ┊ ┊ ┊ ┊ ┊ 订 ┊ ┊ ┊ ┊ ┊ 线 ┊ ┊ ┊ ┊ ┊ ┊ ┊ ┊ ┊ ┊ ┊ ┊ ┊
安徽工业大学 毕业论文
三、结论
(1) 神经网络具有知识和信息的分布式表达、大规模并行处理、自动获取, 知识处理的自适应性、容错性和联想记忆能力等特点。传感信息一般都具有模型难以确定、非线性强的特征, 给被测量的准确获得带来极大困难。神经网络正好迎合了这些特征, 可以完成输入模式到输出模式的复杂映射。所以传感器与神经网络的结合是技术发展的必然。本文提供的将神经网络用于传感信号处理的原理和方法, 对于提高传感器的性能, 挖掘传感器的潜力具有重要作用。
(2) 需要加以说明的是在工程实践中会碰到一些用传感技术手段较难检测甚至不能检测的过程变量,这时, 基于神经网络的软测量技术是解决问题的有效方法。它是依据生产过程中有关的过程变量间的关联, 以一些能够用传感技术检测的过程变量和相应的数学模型, 通过神经网络估计难检测的“传感”技术。
共 44 页 第 44 页
本文来源:https://www.wddqxz.cn/cc0434da9d3143323968011ca300a6c30c22f1f0.html